Owing to the rapid growth of and reliance on data science systems, effectively governing and managing them has become a top priority for both practitioners and researchers. While data science allows us to chart new frontiers, it requires varied forms of discretion and interpretation to ensure the credibility of algorithmic knowledge. Central to this is the notion of trust – how do we reliably know the trustworthiness of data, algorithms, and models?
This is the basis of award-winning research from Cornell Information Science PhD candidate Samir Passi and Associate Professor Steven Jackson, whose paper “Trust in Data Science: Collaboration, Translation, and Accountability in Corporate Data Science Projects” received a Best Paper Award at the upcoming ACM Conference on Computer-Supported Cooperative Work and Social Computing (CSCW), to be held November 3 through 7. The same pair also received a Best Paper Award for “Data Vision: Learning to See Through Algorithmic Abstraction” at last year’s CSCW conference.
“When it comes to data science, people, practitioners, and researchers are faced with an important question: How is it that we trust algorithms that we often don’t understand or can’t explain?” Passi said. “Often, we think of trust in data science as a form of calculated trust, but what we show in the paper is that trust in data science is as much collaborative as it is calculative. The use of numbers such as performance metrics, for instance, isn’t straightforward. Their use depends on the context – who provides numbers, when, to whom, and for what purpose? Numbers have a sort of plasticity to them.”
The paper’s findings came about through on-the-ground fieldwork inside of a multi-billion-dollar technological firm where Passi worked in an unconventional dual role as both data scientist and researcher. Given permission from the company to carry out his research, Passi was offered a detailed look into the challenges corporations face in designing, developing, and using data science systems. The paper identifies four common tensions that challenge collaborative work and understanding within corporate data science projects:
- (un)equivocal numbers – results produced from algorithms do not tell the full story but require discretion and interpretation
- (counter)intuitive knowledge – interpreting algorithmic results requires a delicate balance of intuition and novelty
- (in)credible data – the reliability of data is as much a product of the actors’ lived experience as of technical forms of pre-processing
- (in)scrutable models – teams using data science and algorithms in a corporate setting are using tools that are fundamentally difficult to explain
The paper situates these tensions in two separate projects. On one project, the data science team used a prediction model to help a client marketing company determine how many of its current customers were likely to cancel their paid service, or, in corporate parlance, to “churn.” While both the company’s business and data science teams shared the common goal to minimize churn for their clients, they interpreted algorithmic results differently. The data science team saw the low likely-to-churn probabilities as valuable, while the business team – unclear as to how the figures were even generated – discounted them as incomplete.
Samir Passi and Steve Jackson 2018.jpg
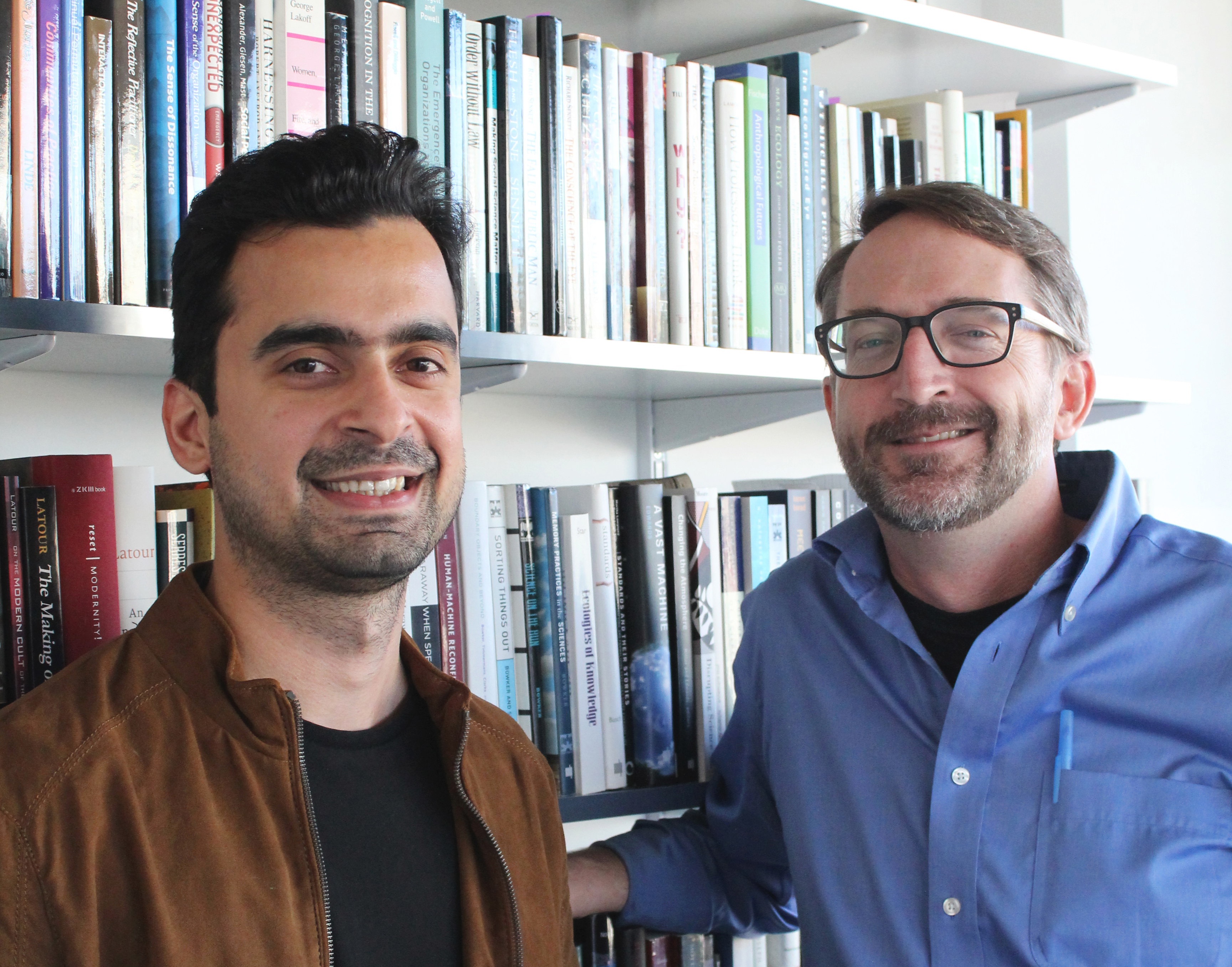
The teams differed similarly on a separate project for a loan financing service, leading the authors to conclude that actors’ trust in data science systems is entangled not only with the perceived credibility of data, but also with their understanding of and confidence in the model’s working.
“Corporate actors prioritize useful results over flawless techniques, working to find pragmatic ways to make the best out of messy situations. They use various strategies to work with, and not necessarily around, doubt and skepticism,” Passi said. “The important point to note here is that real-world applied data science is extremely heterogeneous and collaborative. For instance, we often describe project managers and business analysts as mere users of data science systems, but that is not true. We show in the paper how these experts are also in-part the designers of data science systems.”
Beyond its implications for the present, the paper also connects emerging data science practices to tensions and concerns that go back to the foundations of modern science itself.
“While the paper focuses on challenges and interactions in a given firm,” noted Jackson, “it reminds us that problems of knowledge and uncertainty are alwaysgrounded in a social context–and that attributions of trust are a time-honored and often efficient response to those problems. In many ways, data scientists are now navigating the same concerns that makers and users of experimental science, statistics, and other analytic techniques have negotiated before them. Who and what to trust? Where and how to doubt? And what counts as valid knowledge in a changing and uncertain world?”
Louis DiPietro is the communications coordinator for Cornell's department of Information Science.